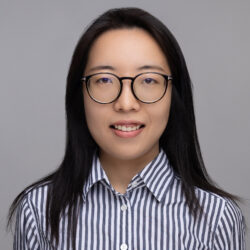 |
    |
Title: Tilted Losses in Machine Learning: Theory and Applications
Speaker: Tian Li, Assistant Professor, The University of Chicago, USA
Abstract:
Heterogeneity not only affects the convergence of federated learning (FL) models, but also poses challenges to a number of other critical constraints including fairness. In this talk, I first introduce a fair federated learning objective, q-Fair FL (q-FFL), to promote consistent quality of service for all clients in the network. Partly motivated by q-FFL and exponential tilting, I then focus on a more general framework to address limitations of empirical risk minimization via tilting, named tilted empirical risk minimization (TERM). I make connections between TERM and related approaches, such as Value-at-Risk, Conditional Value-at-Risk, and distributionally robust optimization, and present batch and stochastic first-order optimization methods for solving TERM at scale. Finally, I show that this approach can be used for a multitude of applications in machine learning, such as enforcing fairness between subgroups, mitigating the effect of outliers, and handling class imbalance—delivering state-of-the-art performance relative to more complex, bespoke solutions for these problems.
Biography
Tian Li will be joining the Department as an Assistant Professor in the Summer of 2024. Her research centers around distributed optimization, federated learning, and trustworthy ML. She is interested in designing, analyzing, and evaluating principled learning algorithms, taking into account practical constraints, to address issues related to accuracy, scalability, trustworthiness, and their interplays. Tian received her Ph.D. in Computer Science from Carnegie Mellon University. Prior to CMU, she received her undergraduate degrees in Computer Science and Economics from Peking University. She received the Best Paper Award at the ICLR Workshop on Secure Machine Learning Systems, was invited to participate in the EECS Rising Stars Workshop, and was recognized as a Rising Star in Machine Learning/Data Science by multiple institutions.
- Code: https://github.com/litian96/TERM
- Blog post: https://blog.ml.cmu.edu/2021/04/02/term/
- Paper: https://www.jmlr.org/papers/v24/21-1095.html
|
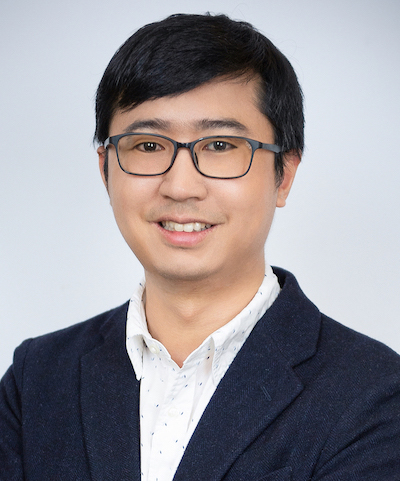 |
    |
Title: Federated Causal Discovery
Speaker: Mingming Gong, Senior Lecturer in Data Science, ARC DECRA Fellow, The University of Melbourne, Australia
Abstract:
To date, most causal directed acyclic graphs (DAGs) structure learning approaches require data to be stored in a central server. However, due to the consideration of privacy protection, data owners gradually refuse to share their personalized raw data to avoid private information leakage, making this task more troublesome by cutting off the first step. Thus, a puzzle arises: \textit{how do we discover the underlying DAG structure from decentralized data?} In this talk, focusing on the additive noise models (ANMs) assumption of data generation, I will introduce our gradient-based learning framework named FedDAG, which can learn the DAG structure without directly touching the local data and also can naturally handle the data heterogeneity.
Biography
I am a senior lecturer in Data Science at the School of Mathematics and Statistics and Melbourne Centre for Data Science, the University of Melbourne (UoM), and an affiliated associate professor of Machine Learning at Mohamed Bin Zayed University of Artificial Intelligence (MBZUAI). I am the co-founder and co-director of UoM Causal Learning & Reasoning Group and the Melbourne Deep Learning Group. Before joining UoM, I was a postdoctoral research fellow at University of Pittsburgh and Carnegie Mellon University, working with Prof Kayhan Batmanghelich and Prof Kun Zhang. I obtained my PhD from University of Technology Sydney, supervised by Prof Dacheng Tao and co-supervised by Prof Kun Zhang, Master degree from Huazhong University of Science and Technology, and bachelor's degree from Nanjing University. From 03/2013 - 10/2013, I was a research intern at Max-Planck Institute for Intelligent Systems (Prof Bernhard Schölkopf's lab).
- FedDAG: Federated DAG Structure Learning
|
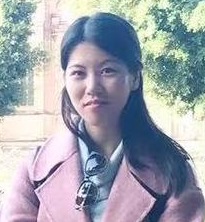 |
    |
Title: When Foundation Model Meets Federated Learning
Speaker: Lingjuan Lyu, Head of Privacy and Security, Sony AI, Japan
Abstract:
Foundation Models (FMs) had received a tremendous attention in the past few years. However, the development of FMs also face a series of bottlenecks such as legal data usage, heavy computation resources, etc. Federated Learning (FL) emerges as a promising solution to address these bottlenecks by allowing training, fine-tuning or enriching FMs by aggregating knowledge from the distributed data sources without direct data sharing, facilitating computation sharing, mitigating the domain gap between training and test data, democratizing the development of FMs, and effectively handling the challenges posed by continuously growing data. Beyond the benefits that FL can bring to FMs, FMs can also greatly contribute to the FL community. In this talk, I will discuss how Foundation Model (FM) and Federated Learning (FL) will interplay and benefit from each other.
Biography
Lingjuan is the Head of Privacy-Preserving Machine Learning (PPML) team in Sony AI. As a globally recognized expert in privacy and security, she is leading a group of excellent scientists and engineers on privacy and security related initiatives across the company. Prior to joining Sony AI, she spent more than eight years working in academia and at industry organizations. Lingjuan received her Ph.D. from the University of Melbourne. She was a recipient of the prestigious IBM PhD Fellowship Award Worldwide. Lingjuan’s current interest is trustworthy AI, mainly on federated learning, responsible foundation model development, data privacy, model robustness, IP protection, on-device AI, etc. She had published over 100 papers in top conferences and journals, including NeurIPS, ICML, ICLR, Nature, etc. She and her papers had won a long list of awards from top main venues, such as ICML Outstanding Paper Award, ACL Area Chair Award, CIKM Best Paper Runner-up Award (only 1), IEEE Outstanding Leadership Award, and many best paper awards from AAAI, IJCAI, WWW, KDD, etc.
- When Foundation Model Meets Federated Learning: Motivations, Challenges, and Future Directions
- TARGET: Federated Class-Continual Learning via Exemplar-Free Distillation
- Taming Heterogeneity to Deal with Test-Time Shift in Federated Learning
- Towards Fair and Privacy-Preserving Federated Deep Models
|